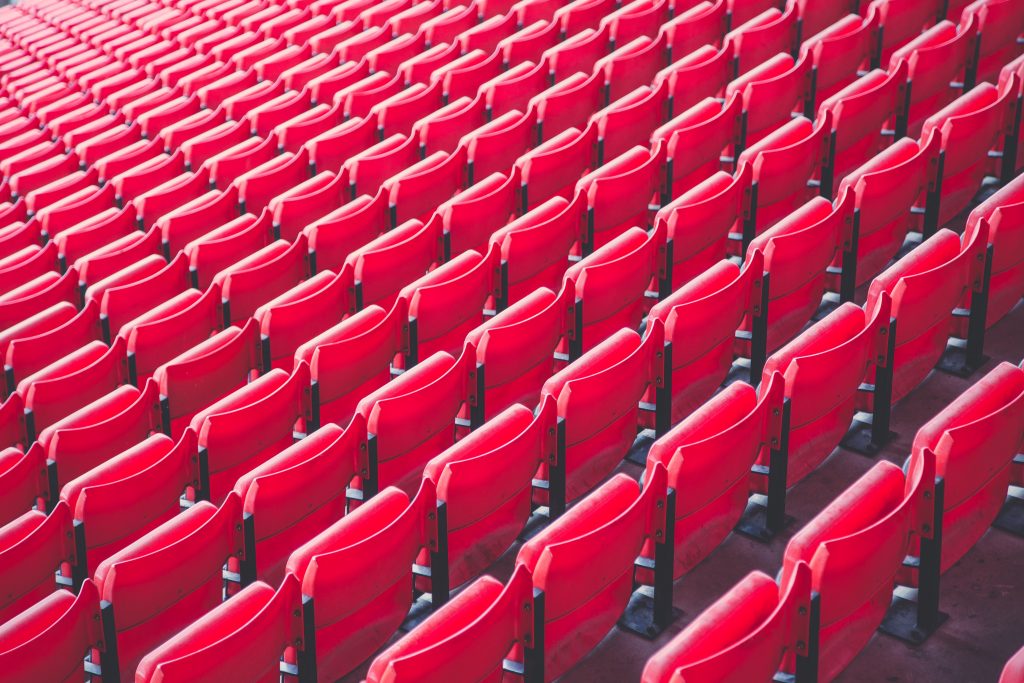
Recently, a teacher of mine (greetings) and me discussed the possibilities through data in basketball after watching a video (How data transformed the NBA | The Economist). Later, I was thinking about how my own point of view and perception of football changed during the last years. On the one hand, there are now many more offers to provide around numbers in professional football than there were some years ago. On the other hand, after reaching my degree in mathematics, I am now working in the field of data analysis and data science for a couple of years. This has further strengthened my analytical view for various topics in general. Has the way I consume football changed during the last years?
Back in the days
When I was a kid, I loved to study football statistics. Every summer, my father bought the kicker special edition which provided information and summary about the past season as well as the coming season.
At the end of the magazine there were several pages of facts and figures, historical numbers, summaries and so on. I learned everything by heart. This still helps me when participating in a football pub quiz (fingers crossed that this continues in post-covid times), since there are many things like records which haven’t changed since then.
Some information could be directly included in upcoming games. Which player has given his teammates many assists? Which team was particularly strong on the counter or in standard situations? Who was particularly confident in scoring penalties? I was always particularly proud when the commentator mentioned a fact or some stats I already knew.
Big Data on the arise
The possibilities of information a commentator provided during the game extended at that time. Before, numbers like the total shots or number of corners were mentioned. With the start of systematic data collection, further key figures were collected during the game and are used for analysis during and after a game. For example, the distance covered by each player and team is often mentioned to analyze the engagement. Meanwhile, more sophisticated KPIs have been developed.
The most famous one is the expected goal (xG) which developed from a marginal phenomenon on specific twitter accounts to a standard metric which each goal is enriched given a typical broadcast. Roughly spoken, it measures the goal probability of a shot by a player, based on similar situations in past games. The sum of expected goals for each team is a measurement of the theoretical success: In how far could each team create the better chances? Based on this idea, further metrics have been developed like expected assists (xA), expected goals against and much more.
During the world cup 2014 I was invited to a discussion on the possibilities and limits of the use of data during a soccer broadcast. With the sports journalist and former Sportstudio– host Michael Steinbrecher, me and some other football enthusiasts discussed this topic from a viewer’s point of view. The outcome was that the amount of information that can be fed to a television audience is somehow limited. This is because a football broadcast is not only addressed to a couple of nerds but to a wide audience. At a certain level, more information displayed can also be disturbing. We agreed that the potential desire for additional information can be satisfied through an additional channel, for example a second screen. The offer for this only needs to be further expanded.
I think that’s exactly what happened in the last years. This does not necessarily require a second screen. For example, SKY offers a so-called Scountingfeed for each match on Saturday evening. The basic idea is to make it possible to observe the whole field like when you are sitting in the stadium. Furthermore, additional data is provided and constantly updated. The demand for this service seems to be high. I found an article from which one can derive that nearly ten percent of the viewers take this opportunity.
Metrics, Metrics, Metrics
Besides the described metrics like xG, there are many metrics and visualizations which are intuitive. For example, the distribution of attacks over four areas. One gets an impression, which side each team prefers for offensive actions. Left outside, left inside, right inside, right outside. An aspect that has been talked about after every game for many decades is thus quantified.
However, there are metrics and models which you have to deal with in advance. Recently, Bundesliga Match Facts by AWS developed a model called Skill-Score. The initiator score measures the impact each player provides regarding the creation of game-changing situations (which means goals). The finisher score not only counts goals but also takes the efficiency into account. The sprinter score measures speed and engagement in sprints. Last, the ball winner score should quantify the ability of a player to cause ball losses to the opposing team.
This is a cool feature, since it provides a measurable number for the impact each player has. But even if it only consists of a weighted combination of already existing KPIs like expected goals, expected passes or speed measures. I doubt whether normal viewers can and want to follow this logic. A commentator also cannot be sure if all viewers can follow if he includes these metrics in the analysis.
A personal view
Personally, I prefer watching a game live in the stadium. But of course, I watch more games on TV than in the stadium. In both cases, I like to think about the potential result and specific challenges for each team in advance. After a game, I often collect several key figures and like listening to analysts and experts. But even though I am a person who views a lot through analytical numerical glasses, I do not consume additional data during the game. Neither in the stadium with the help of my smartphone nor in front of the television. I can’t tell exactly why this is the case. I would say about myself that I have a good understanding of the game and its tactical implications itself. I can describe and understand the structure of a game just taking place without the help of a lot of numbers. It is also my belief that statistics cannot tell the whole story. For sure, I’m not always right in my perception of it either, at least for the moment. But maybe I simply don’t want to rationalize a game completely down to numbers. The passion for the game is far too great for that.